Predictive Maintenance: Too good to be true?
You might have heard this term thrown around as the golden standard of asset management. A lot of people talk about predictive maintenance as if it were some magical solution that will solve all your equipment woes. While far from magical, this high-tech solution does enable some pretty incredible benefits for your company, with use cases that range from manufacturing to healthcare.
Let's start by defining predictive maintenance
Predictive maintenance uses data analysis to monitor equipment and processes in order to detect anomalies in industrial applications that will lead to an issue. Continuously monitoring the equipment and collecting data enables maintenance teams to identify patterns and trends that can be used to predict when equipment is likely to fail. This information can be used to improve maintenance schedules, optimize equipment performance, and extend the life of your equipment. Here, we’ll discuss the pros and cons of implementing predictive maintenance technology solutions and offer up some best practices and use cases.
Predictive maintenance is the asset management practice of repairing an asset or piece of equipment before it fails based on data received about it.
IBM
When investing in a solution like this, companies must ensure that money and personnel are used most effectively and will produce enough return to make it worth it. The U.S. Department of Energy has found that, in many cases, using predictive maintenance can result in an ROI of up to 10 times the cost.
Predictive Maintenance: Advantages & Disadvantages
Like most too-good-to-be-true technology, there are great reasons to implement a predictive maintenance solution. But there are also plenty of challenges, which we’ll elaborate on later.
Why companies invest in predictive maintenance:
Predictive maintenance has several advantages over traditional reactive maintenance.
Increased equipment uptime: A big advantage of predictive maintenance is increased equipment uptime. By predicting when potential issues will occur, maintenance can be performed when most ideal for operations, preventing unnecessary downtime. For example, a manufacturing plant that invests in infrared imaging to read temperature levels in a machine can identify the risk of various components overheating, and action can be taken to prevent machine breakdown. Taking it a step further, machine learning algorithms can learn overheating patterns that have led to machinery breakdowns in the past and can, via an AI model, predict when the equipment is likely to go down if maintenance is not done.
Cost savings: Predictive maintenance can also result in cost savings. Reducing the need for reactive repairs and minimizing equipment downtime can help to lower maintenance costs and increase overall productivity. Additionally, by automating the maintenance process, it can also help to reduce labor costs.
Improved safety: Another advantage of predictive maintenance is improved safety. Identifying and addressing potential safety hazards before they occur can help prevent accidents and injuries, saving a significant amount of money and improving a facility's overall safety.
Increased efficiency: Predictive maintenance can also increase efficiency by automating the maintenance process and identifying issues early. This can help to streamline maintenance operations and reduce the amount of time and resources that are required to maintain equipment. Additionally, by collecting and analyzing data on equipment performance, it can help to improve future maintenance and equipment design decisions.
Extended lifetime of equipment: Predictive maintenance extends the life of equipment by identifying potential problems early on and performing maintenance before a failure occurs. With regular monitoring and maintenance, equipment is kept in optimal condition and its lifespan is extended.
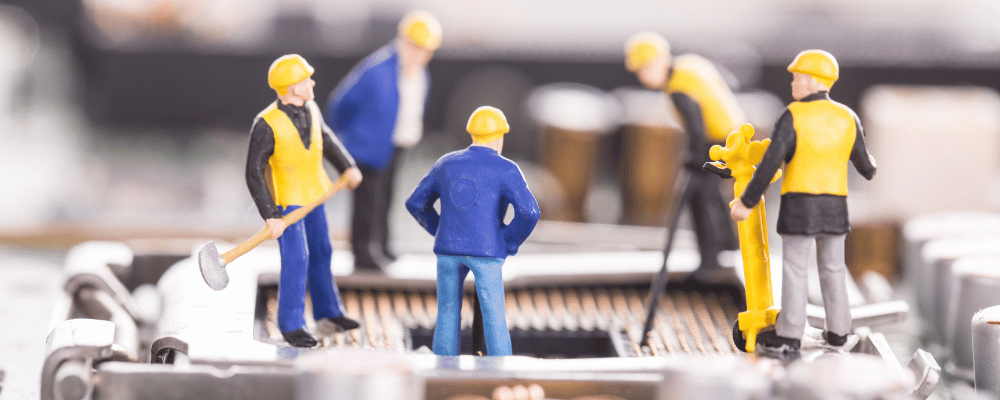
Challenges companies must overcome to reap its benefits
A predictive maintenance solution is a powerful tool that can provide many benefits but also has some challenges.
High costs: Implementing a predictive maintenance program can be expensive. While most companies have much of the equipment, sensors, and software it takes to monitor their equipment, the technology, data aggregation, testing, data scientists, and new processes to turn monitoring into predicting can be costly.
Complexity: Predictive maintenance requires significant data and analytics, which can be complex and difficult to understand for those unfamiliar with the technology. This can make it difficult for maintenance teams to use the data and take action effectively.
Data accuracy: Predictive maintenance relies on accurate data, but data in the field is easily corrupted or incomplete. Often sensors get disconnected, batteries die, the historian databases get full and stop recording, or data was gathered but never annotated, making it impossible to identify events linked to data timestamps.
Limited expertise: Predictive maintenance requires specialized expertise and knowledge in the fields of data science, analytics, and machine learning. If a company lacks the expertise in-house, it can be expensive to hire the necessary experts.
Long implementation time: The time it takes to implement a predictive maintenance solution can vary depending on a number of factors, such as the complexity of the equipment, the amount of data that needs to be collected and analyzed, and the availability of resources and expertise. In general, implementing a predictive maintenance solution can take several months to several years, especially if you’re not using “out-of-the-box” solutions.
Best Practices When Implementing a Predictive Maintenance Solution
Here are some of the predictive maintenance best practices we’ve found to hold true:
Start with a clear understanding of the equipment and its maintenance requirements
Use sensors and other data collection methods to gather accurate and relevant data
Ensure you have event data linked to your real-time sensor data so you can link maintenance and repair events with data that would otherwise make no sense during normal operations
Use advanced analytics and machine learning techniques to analyze the data and predict equipment failures
Prioritize and focus on the most critical equipment first
Continuously monitor and update the predictive models to improve the accuracy
Use a combination of both human and technology-based inspection to validate predictions
Use the insights to create a proactive maintenance schedule
Continuously monitor the results and adjust the plan as needed
Train the maintenance team on predictive maintenance techniques and best practices.
Have a robust maintenance management system in place to track and report on the performance of the equipment
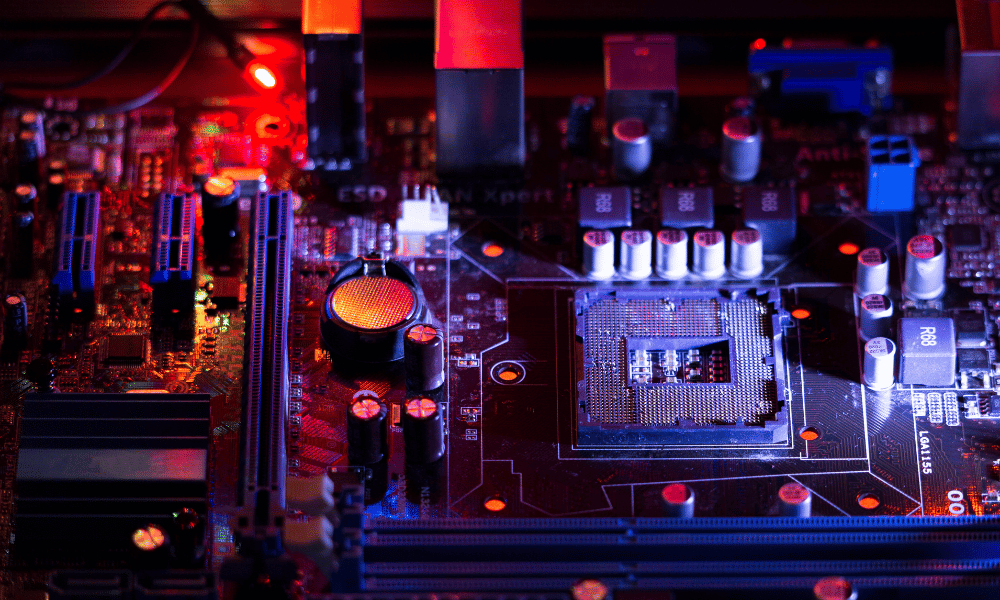
A Quick Study of Use Cases in Different Industries
Here are just some of the ways we apply this smart maintenance tech to different industries.
Predictive Maintenance for Oil and Gas
Oftentimes, oil and gas companies have their equipment in locations that are not easily accessible, for instance, an oil rig located up to 100 miles off-shore. Instead of making routine visits to check on machine health, technicians can use predictive maintenance to determine when they actually need to make repairs.
Predictive Maintenance for Hospitals
Predictive maintenance can be applied to a wide range of critical equipment and systems in hospitals that must avoid miscalibration and downtime at the cost of human lives. There are a wide range of medical devices and equipment, from imaging equipment to ventilators and surgical equipment that must be kept in good working order and available for patient care—possible when predictive maintenance algorithms schedule regular maintenance and repairs.
Predictive Maintenance for Manufacturing
Machine failures and unnecessary equipment and process downtime in manufacturing plants can be very expensive to repair and put a halt in their manufacturing process. Technology such as infrared imaging and vibration sensors are used to predict when a piece of equipment will need to be repaired or replaced.
Predictive Maintenance for Wastewater Treatment Plants
Pumps are a critical component of the wastewater treatment process, and their failure can lead to costly downtime and disruptions to the treatment process. Predictive maintenance can be used to monitor the performance of pumps in real time, using sensors to gather data such as vibration, temperature, and pressure.
Conclusion: Predictive Maintenance Is the Future
Predictive maintenance is like having a crystal ball for your equipment. It allows you to predict when something is about to go wrong and fix it before it actually happens. Imagine never having to deal with a surprise equipment breakdown again! While predictive maintenance has advantages and disadvantages, keeping your machines running smoothly, increasing asset longevity, and minimizing costly downtime definitely make this a worthy solution.