The oil and gas industry is going through a major transformation with the use of powerful computers, advanced machine learning algorithms, and vast amounts of data from various tools. Machine learning is now being used to tackle complex challenges that were previously too difficult for traditional methods. These ML tools can analyze detailed log data and all relevant information, providing a comprehensive approach to solving problems.
Although machine learning tools have their limits, they aren't restricted by the assumptions of analytical solutions or the specific data needs of numerical simulators. By blending ML techniques with analytical models and real-time simulations, the oil and gas industry can address many challenges in upstream and midstream operations, such as optimizing production and reducing equipment downtime.
Oil and gas operations produce a lot of data every day through SCADA systems. To make sense of all this information and work more efficiently, we need to use machine learning and big data techniques. As the industry grows, these technologies are key to driving innovation and achieving top-notch performance.
Here are four machine learning use cases and their benefits:
Real-time production back allocation
Artificial lift system predictive production issues
Hydrocarbon emissions or leak detection
Gathering network flow assurance
1. Real-time production back allocation
Knowing what each well is producing, and how it is producing, is critical to maintaining production and planning well interventions when necessary. Typically, an individual well measurement is costly, so the industry standard is to perform a backward distribution of production from the final custody transfer meter to the producing wells, using the latest available well test information and a mass balance of daily production averages.
However, in addition to being cost-intensive, this process can easily introduce errors in the estimation of the production per well, risking the dynamic information of the process.
It’s necessary to perform dynamic modeling of the production system using physical models parametrically adjusted with machine learning techniques. Based on these models, you can perform a real-time mass, energy, and momentum balance to obtain production per well in real time and the pseudo-dynamic behavior of the production.
Essentially, this means that any events and perturbations in the process are captured. Once this model is built, it can be used to optimize artificial lift systems in real time, find flow assurance problems in real time, and generate predictive recommendations for well intervention.
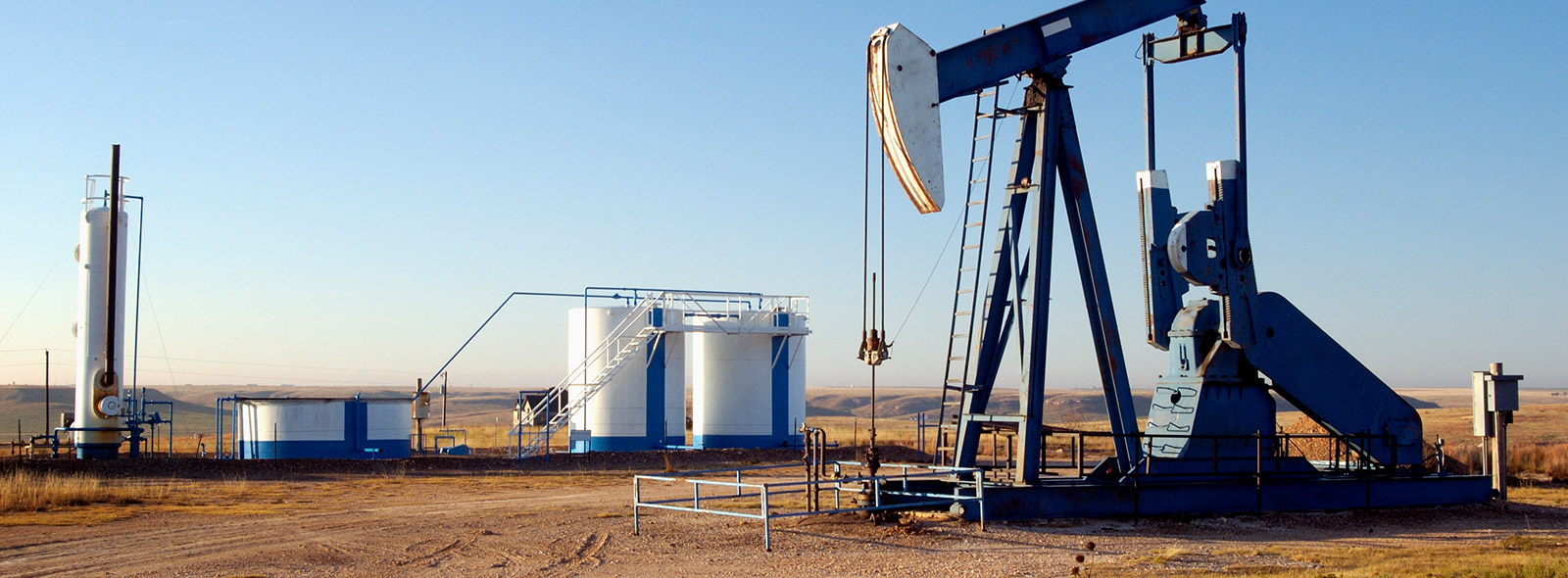
2. Artificial lift system predictive production issues
Maintaining artificial lift systems at optimum performance is not easy due to changing reservoir and surface conditions. Additionally, the high number of wells the operator has to service forces them to prioritize the most productive wells over the less productive ones when allocating limited operating resources. In general, the industry has adopted the ad hoc 80-20 rule to service the 20% of the most productive wells that deliver 80% of the production.
This leaves significant improvements to be had in the remaining 80% of wells if automated systems were in place to monitor them without requiring additional resources. A 12% improvement in these wells would mean almost a 10% improvement in asset production.
Predicting operational problems in real time is possible by applying machine learning and real-time simulation techniques to the data that operators currently have in their SCADA systems about their operations in real time. Once you can predict an event, you can also generate automatic notifications to operators for just-in-time intervention. Now your operations that were reactive and consumed too many resources become the exception, and it is possible to service the entire well with the same resources.
3. Hydrocarbon emissions or leak detection
Oil and gas field operations involve working in areas with a potential risk of hazardous atmospheres, such as H2S levels, which can be fatal to humans. In addition, new environmental regulations regarding the measurement and mitigation of methane emissions are creating new challenges for operators to comply with these regulations with the least possible impact on the profitability of the business.
It’s possible to predict operational problems in real time by applying machine learning and real-time simulation techniques to the data that operators currently have in their SCADA systems about their operations in real time. Once you can predict an event, you can also generate automatic notifications to operators for just-in-time intervention. Now your operations that were reactive and consumed too many resources before become the exception, and it is possible to service the entire well with the same resources.
.jpg)
4. Gathering network flow assurance
Sand plugging, hydrocarbon precipitation, hydrate formation, or back pressure due to slugging, among others, can create havoc on surface production transportation networks. And no one wants that, especially considering how complex these systems are and the implications of production losses.
Knowing the real-time pressure profile at every point in the transportation network, as well as the pseudo-three-phase composition, allows you to predict undesirable events and act proactively to mitigate or eliminate production loss.
Real-time SCADA data together with machine learning techniques applied to physical models with parametric tuning enables the generation of virtual three-phase flow and pressure measurement—at any point of the above-ground production network—making it possible to generate automatic alerts in case of any flow assurance event.
The ROI of Implementing Data Analytics and Machine Learning in the Oil and Gas Industry
In the oil and gas industry, the perceived value of machine learning is undoubtedly high, but its application poses significant challenges. The dynamic nature of O&G operations, characterized by constant changes such as new wells and interventions, presents obstacles to seamless ML integration, resulting in the need to navigate complex operational processes while ensuring the continuity of operational information systems.
The challenges of implementing ML, or MLOps, go beyond managing process changes and include scalability and maintaining model accuracy in a constantly evolving production environment. Reservoir behavior and production dynamics are inherently changing, making trained models obsolete in a short time unless they are implemented with automatic retraining rules and validation with field measurements.
Despite these challenges, the potential benefits of machine learning implementation are compelling. At Timbergrove, we bring a wealth of experience to overcome the obstacles and unleash the transformative power of data analytics and ML in the O&G sector.
For customized information on how your organization can take advantage of machine learning models in the oil and gas sector, let's start a conversation today.